About Yalo: Yalo is a conversational commerce software company that allows businesses to connect with their customers and enhance their outreach and sales strategies through personalized conversation flows in messaging apps. Yalo’s platform is powered by GenAI technology and fueled by petabytes of data. It played a pivotal role in transforming business communication for clients like Buyer, PepsiCo and others.
The Yalo data engineering team ingests petabytes of data each month, which allows them to innovate and drive business growth. However, this comes with the need for significant computational resources. In this case study, Clarisa Castelloni, Analytics Manager at Yalo, will share how they managed to develop a framework that reduced their BigQuery costs by 30% within a remarkable 40 days following the project’s launch.
Challenge: By mid-year, it was clear that due to the rise in consumption and an increase in Google BigQuery, Yalo’s costs had spiked by nearly three-quarters. While the increase in consumption was anticipated due to the onboarding of new clients and the utilization of more GBQ capabilities, the Yalo team still wanted to ensure that every dollar invested in data platform was driving the business.
Unfolding the challenge. Yalo’s Costs Framework.
Clarisa Castelloni, Analytics Engineering Manager at Yalo, took on a project to build a framework to optimize Google BigQuery costs and everything related to the data team to drive efficiency with every dollar used within their data platform.
As she says, “I’m on a mission to raise cost consciousness.”
To understand what’s driving the spike the most, Clarisa and her team developed a framework with a top-down approach. They started from the basics – Google Cloud Console Billing. Yalo team could see that over 50% of their cloud bill is driven by the Analytics & Data team.
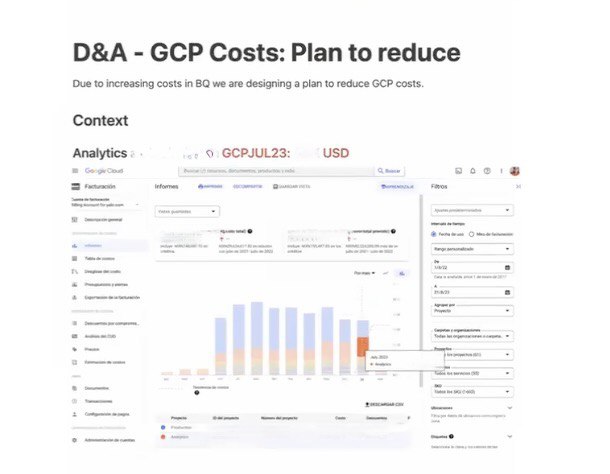
For the second step in analyzing the Analytics & Data team’s spending, Clarisa conducted a detailed breakdown of the costs incurred by these teams, categorizing them by the services used. This analysis was crucial in identifying which solutions contributed the most to overall expenses. Notably, it was found that GBQ accounted for over significant majority of Yalo’s Google Cloud bill.
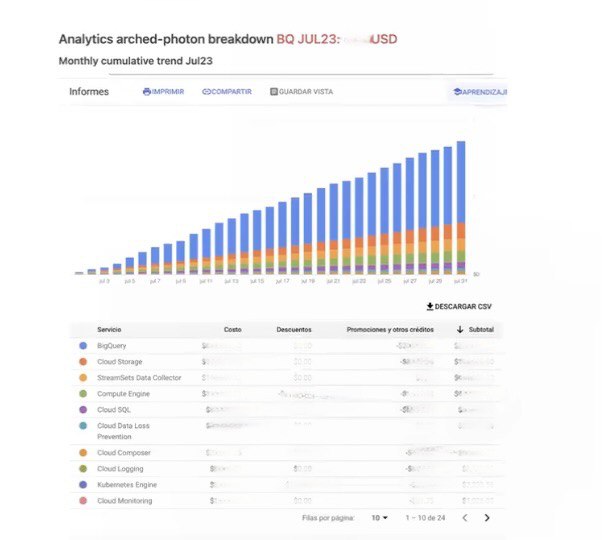
The third step was to drill down into GBQ consumption and identify which users and service accounts were driving the most usage. Additionally, the Yalo team identified the most costly queries on their data platform.
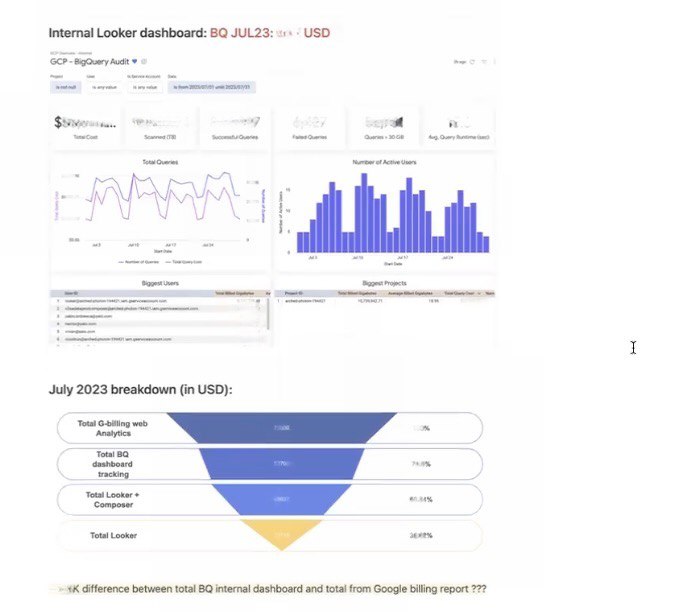
This analysis helped to understand that the highest consumption was driven by Looker and Cloud Composer. Author’s note: surprise, surprise.
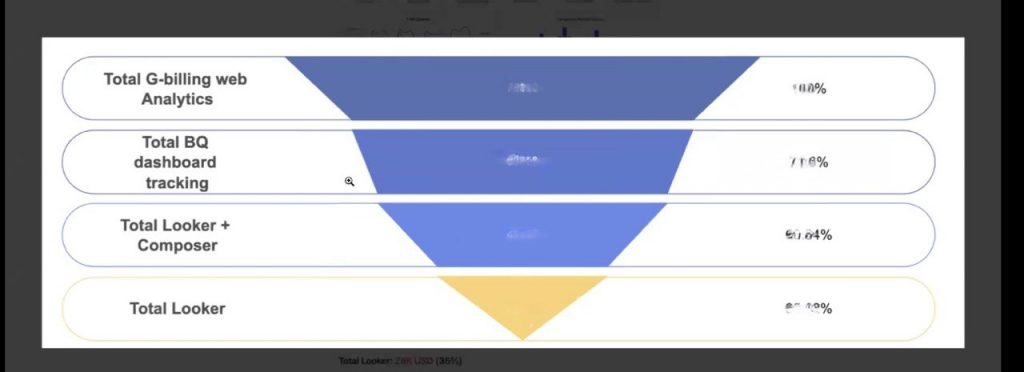
This analysis helped Yalo understand that Looker and Cloud Composer were responsible for well above three-quarters of Google Cloud Costs and more than half of Google BigQuery costs alone. These two services drove much of the Google BigQuery compute usage.
The fourth step in this cost analysis was to break down the compute costs by users and service accounts.
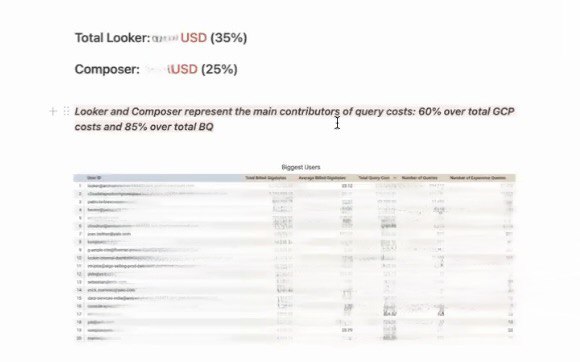
The final, fifth point of the cost analysis was to identify how much the individual pipelines connected to Composer and Looker cost so the team could work on optimizing them and improving performance. This is where Masthead helped the Yalo team gain visibility into the pipeline costs, their frequency, their source tables or data sources, and their owners. All of this was grouped by the technology used.
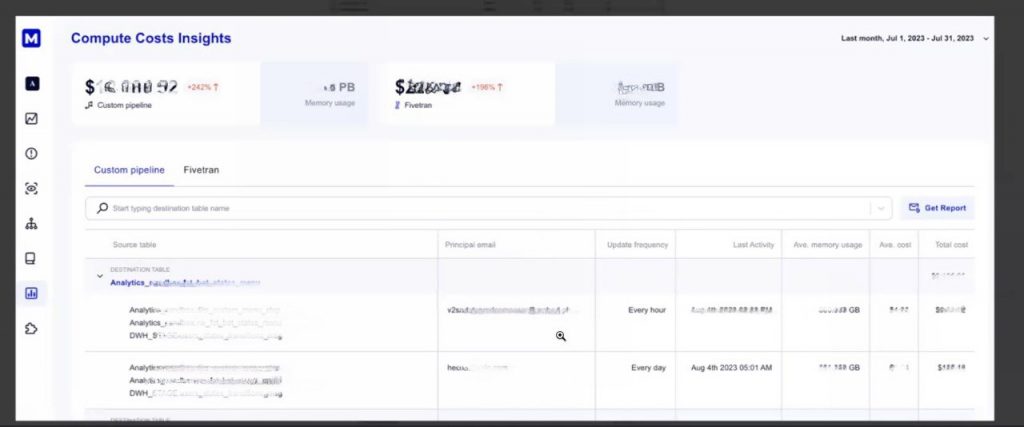
That epiphany moment for the Yalo team came when they were able to see how much each pipeline cost over the chosen time frame and the frequency of their updates.
Following that, the Yalo team was able to download a full report of all the jobs running in their Google BigQuery project, ordered by pipeline size and costs.
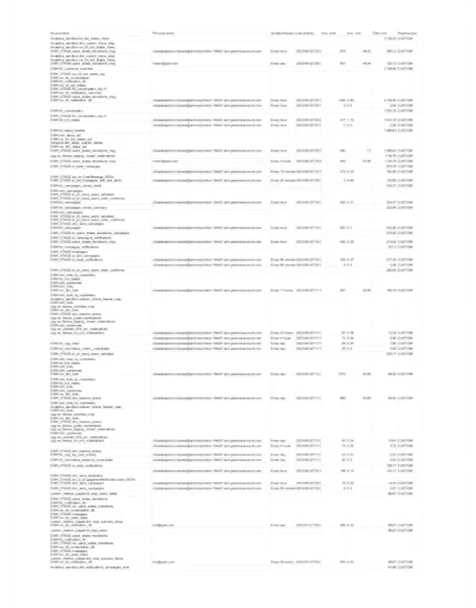
After an in-depth analysis, the hard work began. As soon as the Yalo data team identified the most costly processes, Clarisa introduced the “Demeter Plan.” The idea behind it was simple yet effective:
- Reduce the frequency of pipelines, for example, from a 1-hour to a 2-hour update.
- Ensure that all pipelines operate in such a way that tables are partitioned and clustered.
- Review all pipelines costing over $100 to optimize them with best practices, ensuring that code is written with cost consciousness.
- Identify and delete all deprecated procedures.
- Identify and delete all obsolete and unused tables.
“The idea was simple: if we have a pipeline that runs every hour and costs us around $500, it should not be happening. We took care of those first,” said Clarisa Castelloni.
Results
The instant value provided by Masthead was significant—it surfaced a number of obsolete pipelines that had not been in use for a long time. Another important dimension was the frequency of pipeline runs in connection with their costs. This enabled the Yalo team to cut the costs of some pipelines and models by reducing their run frequency.
“Working with Masthead enabled us to optimize frequency, improve code efficiency, and deprecate orphan pipelines. As a result, the Yalo team was able to cut their GBQ costs by 30% within a month of starting the project.” Clarisa Castelloni.
What’s next for Yalo?
Cost control practices are ongoing work. The Yalo data team constantly tests and implements new data solutions in their stack while working with data consultants beyond their in-house team. Masthead helped the Yalo team proactively understand how newly adopted data solutions contribute to their Google Cloud bill and to draw conclusions: Are they being used effectively, and what is the true total cost of ownership for these new solutions in Yalo’s data stack? It also enabled them to assess the efficiency of practices introduced by outsourced data team members, providing timely feedback that aids in controlling GCP costs.
As usual, technical tasks seem difficult, but securing buy-in across the organization is even harder. The Yalo team realized that individual queries could be unpredictably expensive and that data literacy is a priority they are now addressing beyond just the data team.
If you have any questions on how to build up the cost-saving framework for your organization, please feel free to reach out to Yuliia to jam some ideas together.
Learn more about the BigQuery and Masthead Data partnership.
Learn more about Masthead Data.
To learn more about Masthead Data’s marketplace offerings.
To learn more about Google Cloud Ready – BigQuery.