Disclaimer: The thoughts and observations expressed are my own. They are based on numerous discussions with Google Cloud Product team members, users, champions, and partners, as well as a few attended talks.
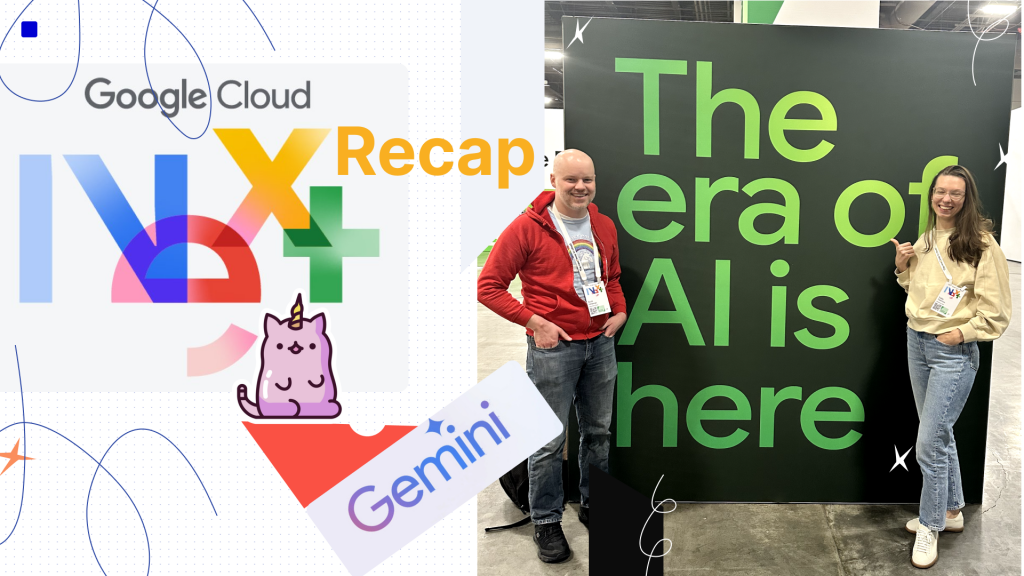
All the splash at Next’24 in Vegas was about Gemini’s most powerful model and Google proving they are a leader in AI. The new Gemini miracle is now available in almost all Google Cloud products. To make this happen, the GCP team did a tremendous job launching an AI infrastructure that can support supercomputer architecture.
For me, the interesting angle with Gemini is how it integrates with enterprise data and search. This means that GCP enterprise customers can relatively effortlessly take their pre-trained models loaded with business data and combine them with Google search data in their own environments.
To be honest, I’m skeptical about combining business data with search data, but the ease of applying AI to enterprise internal data inspires me. In practice, this means organizations can connect AI models to either AlloyDB or BigQuery.
While AlloyDB has not yet achieved widespread adoption, BigQuery is being largely promoted as a central piece of the data ecosystem within organizations to extract value from the latest Google AI innovations. In other words, Google BigQuery is becoming a fundamental data platform for organizations. I do not think that Google BigQuery’s role was ever underrated within Google Cloud (Alphabet). The Information estimates that BigQuery generated around 80% of GCP’s revenue in 2021. I would be more conservative in my estimations and suggest about 50%, but it’s still a tremendous success for the product.
Yeah, but what that actually mean for the Data teams
I mentioned in the Next and latest release notes that the GCP team is making a big bet on BigQuery and elevating it more than ever. (I’m okay with being wrong in my judgment here; let me know if you see this differently).
To prove my point, I mentioned a few things that Google BigQuery has done, which were absolutely unimaginable a few years ago.
They recently released a direct connector from Salesforce and Facebook (👀). With Salesforce, they went a step further and developed a connector to push data back to Salesforce. (I don’t expect that level of privilege for Facebook, but you never know ¯\(ツ)/¯). Moreover, the connector to Salesforce is one of the reasons a lot of data connector vendors exist out there… Of course, they made it as easy to use as the GCP team possibly could.Furthermore, a few new connectors to BigQuery are on their way, including ones for Workday, Hadoop, Confluent, and JIRA.
Another sign that Google BigQuery is becoming a central piece in clients’ ecosystems is the announcement of Apache Kafka for Google BigQuery. A shout-out to the Google Cloud team for the naming (haha)! But this also proves my point that now, everything is for BigQuery. A couple of years ago, they were overlooking growing businesses like Aiven, which provisioned and managed Kafka for organizations, focusing instead on their own event streaming technology, Pub/Sub. Now, Kafka is readily accessible and easy to enable in the GCP console.
The second part of elevating Google BigQuery involves impressing data analysts, data engineers, data scientists, and other users with little to no SQL knowledge through a collaborative and exceptional user experience.
Sure enough, you’ve seen BigQuery expansion to BigQuery Studio, where several features were added to foster a collaborative experience—like the SQL editor, saved queries in Dataform, job history, and data canvas. A lot of effort was made to ensure high-quality data and its discovery with the latest releases, including Dataplex data profiling and the announcement of column-level lineage. (God, we’ve had it at Masthead for over a year now).
The other part involves the GCP team expanding Google BigQuery to a wider user range by enabling Python notebooks, BigQuery DataFrames, and a PySpark editor. This means that users with knowledge of, or a preference for, Python and PySpark can now utilize their skills in Google BigQuery.
I think we’ll see more exciting launches for BigQuery Studio soon, as the strategy to enable AI lies through positioning data correctly and making it easy to use AI on it.
Wrapping up
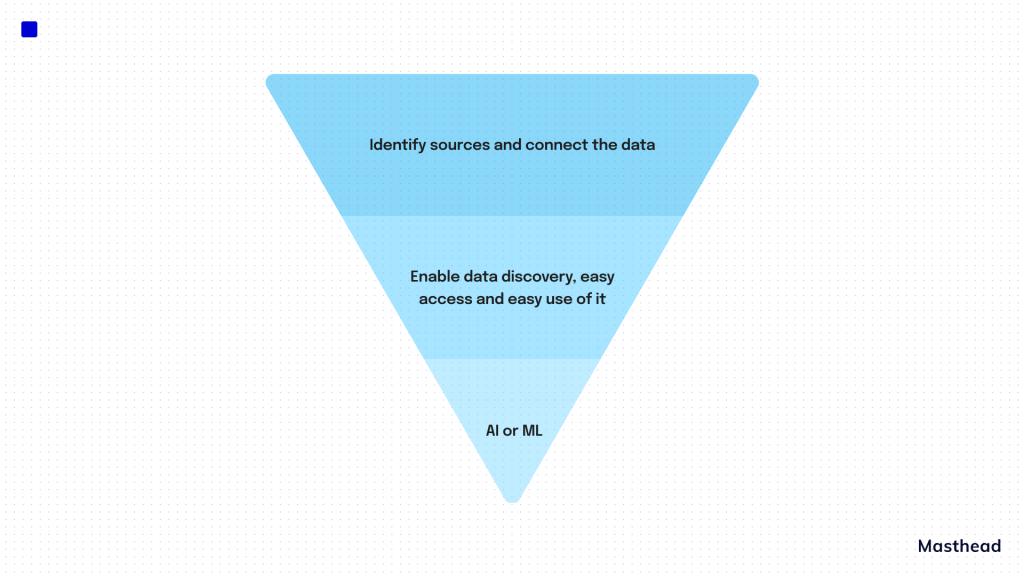
To summarize my thoughts and observations, Google is making a bet by focusing on enterprise clients to help them make sense of their data, but also making it as simple as possible and as they can. To do so, GCP shows the commitment to release functionality in the following areas:
1. Connect and Collect Data: The GCP team is striving to create a seamless and easy experience for enterprise users by consolidating data from different sources into a single place – BigQuery (not necessarily within one project). They aim to simplify how data—from Excel, Facebook ad accounts, or real-time events—appears in Google BigQuery for every employee who has access.
2. Model and Transform Data: This is about making data accessible and usable, regardless of the user’s skills or preferences. Whether it’s SQL, Python, PySpark, or even simple semantic queries, the goal is to enable a broad range of users to access, manipulate, and collaborate on data within BigQuery Studio. Another very expected development is enabling people with no technical skills to ask questions that are converted into SQL queries. However, I’m curious about how many people are using this feature and, more importantly, how many trust the results it generates.
3. Application of AI: If all the components mentioned above are effectively implemented, the organization will be ready to apply AI, and I believe GCP is largely prepared for this transition. However, the question remains: what portion of GCP customers are ready to enable AI, and what are their use cases? Google is doing a tremendous job preparing enterprise clients for this stage, where data readiness is often a hurdle. Now, they are making significant efforts to bridge this gap by positioning BigQuery Studio as the center of their Data Platform. This is very much aligned with what we see working with our clients at Masthead. Many organizations already treat their BigQuery and all the surrounding infrastructure as a Data Platform, and we are very excited about the changes yet to come for the majority of the market.