Despite investing large sums into BigData and AI, 69% of Executive Managers admitted that their companies are struggling to adopt a data-driven culture. Given the amount of resources spent on building data teams, deploying data tools, onboarding employees, and maintaining the infrastructure, the glass looks half empty. Why is it so? What should you consider before leading your company to a data-driven future?
Data science roles have been on the rise over the past 6 years and the shortage of professionals in the market shows a clear trend. Companies, from early startups to fintech giants, are working on leveraging the AI strategy. In short, their goal is to improve their business with AI and ML technologies. The big sin of these companies is hiring a data scientist team, but not recognizing what stage of data adoption they are at. Monica Rogatti presents a hierarchy of data needs:
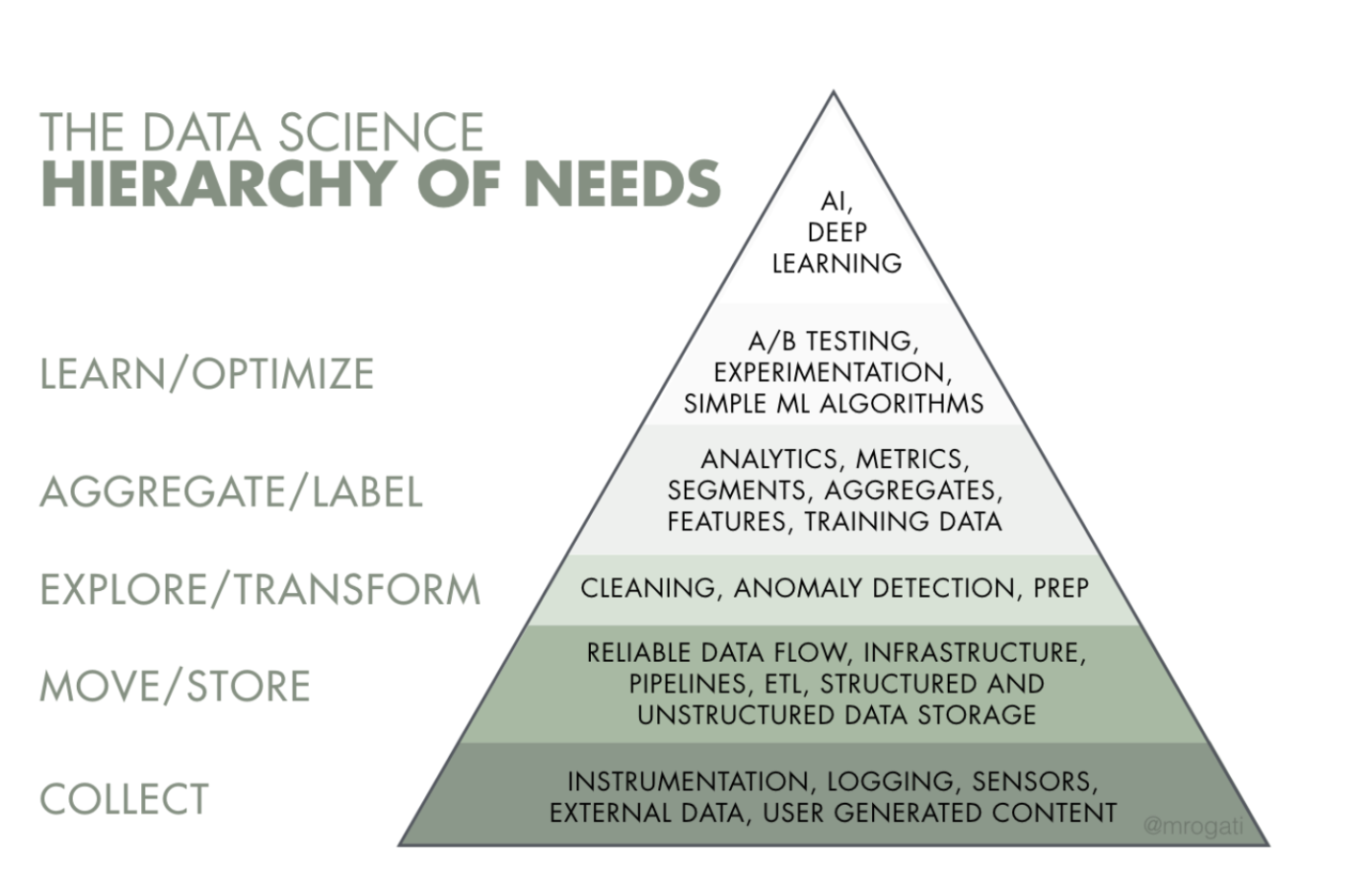
Source: The fantastic Monica Rogati’s post on hackernoon “The AI Hierarchy of Needs”
Unfortunately, few companies realize that merely collecting data does not mean that it takes them to the level where they can apply the ML models. Starting to collect the data also does not mean that a company becomes data-driven.
The above pyramid should not only guide data teams on what level where they should be at to fulfill the company’s data-driven missions.It should also be the resource for the data-culture leader to assess the level of data culture availability in their company.
Another thing I’ve experienced in companies is that a data-driven culture is meant as having a data team (at least one part-timer) and backing up any of the management’s decisions at least with some data. This should be fixed, seriously. Having some data to support your decisions may be better than having no data, but that’s not always the case.
And it’s definitely not enough. Once you, as a decision-maker, have started to use data in your decisions, you need to make sure this data is complete, full, doesn’t have duplicates… the list goes on. It’s only after you have this covered that you can be sure your data is reliable, trustworthy, and can be used for decision making. This is the way of thinking in the company that can power the way to adopting a data-driven culture.
“Quality over quantity” should be an underlying principle for the entire company when it comes to using data.
According to a study by Cambridge researchers, data dispersion remains the main obstacle towards efficient data use and is a widely ignored problem. The problem exists because of the numbers of different data streams coming from different sources, using different data schemas and different conventions, stored and accessed in different ways. To say simply, this is lack of standardization. Solving the problem takes meticulous work of data engineers to combine those vast data sources into a single data set suitable for working with it. Once that is accomplished, the data team starts to check and clean data, which takes around 50 to 80% of their work time.
High data quality takes a lot of effort, time, and investment, which is often skipped towards faster results and often poor quality. This is why clean data is of paramount importance. Without it, the leadership can’t be sure they’re making the right strategic decisions. Once an organization has a dirty data problem, the mess that follows isn’t pretty. There is no silver bullet for adopting a data-driven culture overnight or even over a month.
The support of the C-level management for the data team is a vital part of the journey towards the data-driven culture. While the data team is working behind-the-scenes to make it happen, the business side should help:
- Champion your data team and help to create the buy-in for change from each and every employee.
- Promote the importance of data quality over quantity.
- Help the data team to understand the business’ objectives, and establish North Star metrics to guide your data quality journey.
- Connect data consumers with the data team.
- Work to set first meaningful Data SLA to measure the data quality.
Making a shift towards data culture takes time, effort, and lots of money. Small details can mean the difference between success and a costly failure. There is no easy recipe for getting things done fast. And yet, staying committed to your goal, understanding the process, and taking incremental steps towards a data-driven culture will help a lot.
What’s your experience with data culture? Let us know!